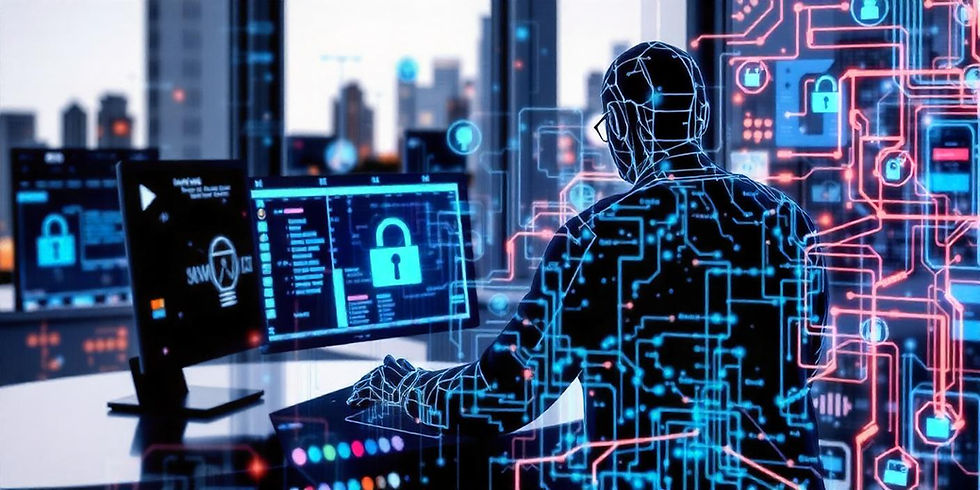
As cyber threats continue to evolve, so must the tools that defend against them. Cybersecurity professionals are increasingly turning to machine learning (ML) and artificial intelligence (AI) to detect and mitigate these ever-growing risks. The fusion of machine learning and cybersecurity has ushered in a new era of proactive, rather than reactive, security measures, empowering organizations to anticipate, identify, and respond to threats more effectively.
In this article, we’ll dive into how AI and machine learning in cybersecurity are transforming the industry, the potential they bring to cybersecurity projects, and how professionals can incorporate these technologies into their security strategy.
Understanding Machine Learning in Cybersecurity
Machine learning is a subset of AI where systems learn from data sets to make decisions without being explicitly programmed. In cybersecurity, machine learning offers enhanced capabilities to detect malicious activities, respond to cyber attacks, and analyze vast amounts of data in real-time. Unlike traditional security measures that rely on pre-defined rules, ML in cybersecurity provides flexibility and adaptability.
For instance, machine learning algorithms can recognize patterns in behavioral data that may signal potential threats. This ability to learn and improve over time makes machine learning an invaluable tool in combating sophisticated threats like phishing attacks and anomaly detection.
Why AI and Machine Learning in Cybersecurity Are Game-Changing
Machine learning's power lies in its ability to analyze large volumes of data quickly and accurately, making it a perfect fit for cybersecurity, where vast quantities of data are collected every second. ML can analyze these massive data sets and detect anomalies faster than human analysts ever could.
Enhancing Threat Detection
One of the primary applications of machine learning in computer security is anomaly detection. Instead of relying on signature-based detection systems, AI and ML in cybersecurity can identify unusual patterns in network behavior, pointing to potential threats. For example, an ML algorithm can detect behavioral patterns such as unusual login times, massive data transfers, or access attempts from unfamiliar locations that could signal a potential threat.
Deep learning, a more advanced form of machine learning, further strengthens cybersecurity measures by using neural networks to predict and analyze threats. This technology excels in spotting hidden patterns that may be missed by traditional methods, improving detection of zero-day vulnerabilities and malware.
Automating Responses to Threats
Another significant advantage of ML in cyber security is the ability to automate responses to detected threats. AI and machine learning can instantly flag suspicious activities and, in some cases, take pre-programmed actions such as isolating compromised systems or shutting down specific network components to prevent further damage.
Automating responses reduces the pressure on security teams, allowing them to focus on more complex tasks. This automation is particularly valuable when dealing with large-scale cyber attacks, where human response times may not be fast enough to mitigate the damage.
Key Machine Learning Algorithms Used in Cybersecurity
Different types of machine learning algorithms are applied across cybersecurity projects, each with its unique strengths. Here’s a look at some of the most widely used ML algorithms in cybersecurity:
Supervised Learning
In supervised learning, models are trained on a labeled dataset, learning to classify new data based on historical examples. This technique is commonly used in identifying known types of malicious activities by categorizing them based on past incidents. For instance, a system might be trained to detect phishing attempts by analyzing past phishing emails and learning to identify similar patterns in future emails.
Unsupervised Learning
Unsupervised learning does not require labeled data. Instead, the algorithm is tasked with finding patterns in the data on its own. This approach is particularly effective for anomaly detection in networks, identifying abnormal behaviors that may indicate cyber attacks or data breaches. Since cybercriminals are constantly inventing new attack vectors, unsupervised learning is crucial in discovering previously unknown threats.
Deep Learning
Deep learning, as mentioned earlier, is a branch of machine learning that uses neural networks to analyze data. In cybersecurity, deep learning models can process vast amounts of data from various sources to recognize patterns that might be missed by traditional security systems. Deep learning and cyber security together enable the analysis of complex data structures, which enhances the identification of subtle, yet dangerous threats.
Applications of Machine Learning in Cybersecurity Projects
Machine learning isn’t just a buzzword in cybersecurity—it has real, tangible applications that improve the security posture of organizations worldwide.
Phishing Attack Detection
Phishing is one of the most common types of cyber attacks, and traditional detection methods often fall short due to the ever-evolving tactics of attackers. AI and ML cybersecurity tools can analyze emails in real-time, comparing them to known phishing patterns and detecting even the most sophisticated phishing attempts.
Malware Detection
Machine learning algorithms are increasingly used in detecting malware. Unlike traditional methods that rely on known malware signatures, machine learning models can identify malicious behavior based on patterns, such as unusual file access or network traffic spikes, enabling the detection of new, previously unknown malware strains.
Behavioral Analysis for Insider Threats
Insider threats are particularly dangerous because they come from trusted employees or partners. However, machine learning security models can monitor user behavior, identifying deviations from the norm. For example, if a user who typically accesses small amounts of sensitive data suddenly begins downloading large datasets, AI and ML systems can flag this behavior as suspicious.
Implementing Machine Learning in Your Cybersecurity Strategy
Integrating AI and ML into your cybersecurity strategy can be a game-changer, but it requires careful planning. Here’s how to start:
Data Collection and Preparation
The success of ML cybersecurity solutions largely depends on the quality of the data used for training the models. It's essential to gather diverse and comprehensive data sets that include normal and malicious activities. This data will help the model understand what constitutes a threat.
Continuous Learning and Adaptation
Cyber threats evolve, and so should your machine learning models. Ensure that your models can continuously learn from new data, adapting to emerging threats. Partnering with data scientists who specialize in machine learning can help fine-tune your models for optimal performance.
Collaboration Between Security Teams and AI Experts
It's vital to foster collaboration between your security teams and machine learning experts. Cybersecurity professionals can provide critical insights into which behaviors should be flagged as suspicious, while AI specialists ensure the models are properly trained and optimized.
The Future of Cybersecurity with AI and Machine Learning
The growing role of AI and machine learning in cybersecurity is undeniable. As cyber threats become more sophisticated, these technologies will play an increasingly critical role in securing networks and data. From automating responses to anomaly detection to improving threat prediction, ML in cyber security projects offers a proactive defense mechanism that can safeguard organizations against future attacks.
Security teams looking to enhance their cybersecurity measures must embrace AI and machine learning tools. These technologies are not just helpful but essential in combating today's advanced cyber threats.
Are you ready to integrate machine learning into your cybersecurity strategy? Explore our solutions at Offensive Security Manager (OSM) to see how we can help protect your organization from evolving cyber threats.
By incorporating machine learning and AI into your security framework, you’re taking a step toward a more secure future. Start exploring how AI-driven solutions can enhance your cybersecurity today.
Click to see how Offensive Security Manager (OSM) can help you with its AI capabilities to prevent cyber attacks.